Landing on Mars, With a Little Help From Artificial Intelligence
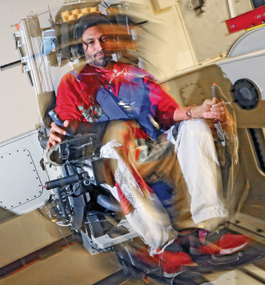
Mike Lovett
Research scientist Vivekanand Vimal, GSAS PhD’17, demonstrates the movement of the Multi-Axis Rotation System chair, which tests people’s balancing skills and spatial orientation.
by David Levin
Imagine yourself on a mission to Mars: For several months, you’ve been cruising through space in cramped quarters. Now you’re poised to enter the Red Planet’s thin atmosphere. Follow the right trajectory, and you’ll land safely. Angle your craft too far in any direction, and you’ll smash violently into a rust-colored hillside. Suddenly, with no warning, your vehicle lurches off course. Can you correct it in time to save your mission?
Questions like this are at the core of Vivekanand Vimal’s work. A research scientist at Brandeis’ Ashton Graybiel Spatial Orientation Lab, Vimal, GSAS PhD’17, studies the human vestibular system, a cluster of tiny structures in the inner ear responsible for balance. Thanks to gravity, the vestibular system makes it possible for us to sense which way is up or down, even with our eyes closed. In space, however, it’s not nearly as useful.
So Vimal and his colleagues are researching ways to help space travelers overcome spatial disorientation using artificial intelligence. If AI can sense when a crash or a loss of control is imminent, says Vimal, it could briefly take control of a spacecraft to nudge it back onto a safe trajectory. In order to do that, though, the system would need to know how specific pilots will react to the motion of their ship.
“Each person is going to experience and respond to spatial disorientation in a unique way,” he says. “These individual differences matter.”
Vimal has been gathering individualized data in the lab by exposing volunteers to a rudimentary Mars-landing scenario. Using a specially constructed Multi-Axis Rotation System chair, which can tip, spin and rotate in all directions, he tilts his blindfolded subjects onto their back and asks them to try to steer the chair with a small joystick. The chair is programmed to act like an inverted pendulum. Without constant correction, it won’t stay at its balance point, and will tip to one side and “crash.”
In 2019, Vimal ran a cohort of 34 people through this wringer, testing their balancing skills in the chair multiple times over two days. The initial results, he says, were surprising. Existing scientific literature had not predicted how badly subjects would perform. Because they had no visual or gravitational cues, all of them immediately became disoriented.
After several attempts, Vimal says, a number of subjects improved, making more controlled, deliberate joystick movements to maintain balance. But some of the cohort actually got worse with practice.
“This was mind-blowing,” Vimal says. “I immediately wanted to know: Why does this happen? Can we predict who might get worse, or when they’ll get worse?”
In collaboration with faculty members Pengyu Hong, Paul DiZio and James Lackner, and graduate students Yonglin Wang, GSAS MS’21, and Jie Tang, IBS MSBA’20, Vimal developed a deep-learning model, a kind of specialized computer software that identifies complex patterns and relationships within sets of numbers.
The software revealed a clear result: In nearly every case, it could predict with 95% accuracy whether a subject would crash 800 milliseconds into the future.
Vimal and his colleagues presented their findings in the journal Frontiers in Physiology earlier this year.
One of the study’s biggest achievements, Vimal says, is that its predictions were based on data from an extremely small number of subjects — a technique that could prove especially useful for the spaceflight community, where data come from only a handful of astronauts.
“Usually, with deep learning, you’re dealing with data from tens of thousands or hundreds of thousands of people,” Vimal says. “We want to make a general artificial intelligence that can learn from data collected from only a few astronauts. It will need to take really noisy, bizarre, anomalous data, where a pilot may be doing the weirdest stuff imaginable because they are disoriented, and use that data to predict when something really bad — like loss of control or crashing — is going to happen.”
For Vimal, the most exciting aspect of space exploration is the uncertainty. “We have no idea what the perfect solutions are and, therefore, can’t train AI beforehand,” he says. “Instead, we have to innovate a new partnership between humans and AI, where they learn together.”
Although it’s a long way from being used in actual spaceflight, Vimal’s work could have more immediate applications here on Earth. People with severe vertigo, for instance, can sometimes have trouble staying upright and walking. If a wearable system could predict which way they’re about to stumble, it might be able to provide other sensory cues to reorient them before they fall.
David Levin is a science journalist in the Boston area.