Connor Zawacki
Miller Lab
Department of Biology
Brandeis University
Inhibition Stabilization: a Framework for Information Processing
An attractor network is a model for a system of neurons whose activity tends towards one of several discrete steady stable states, called attractor states. A network of neurons with a large number of attractor states with comparatively few neurons could provide a plausible framework for information processing.
In the past, attempts to computationally replicate such a network have yielded inadequate results, characterized by problems such as unbiologically high firing rates or requiring the fine tuning of many parameters. There is strong experimental evidence in cortical and hippocampal circuits for system dynamics to follow an inhibition stabilized regime, characterized by strong recurrent excitation which is stabilized by stronger recurrent inhibition.
Use of firing rate models in the inhibition stabilized regime yields more promising results, as multiple attractor states can be stabilized with arbitrary low firing rates. By simulating large variations of connection strengths while fixing other parameters, we can test both the number of possible steady states for a given parameter set, and potentially define a relationship between connection strengths required to produce multistability. We successfully produced several networks capable of a combinatorial explosion of attractor states, increasing exponentially with the number of units participating.
Moreover, this network continued to produce this large number of attractor states for a variety of connection strengths between units, making it a competent conceptual framework for complex information processing or decision making. Among the steps forward is to test if results are replicable in a variety of other parameter sets and connection patterns to confirm how widely applicable this model is.
Personal Statement
Truth be told, at the beginning of this summer, I was anxious, unprepared and unsure that I deserved the funding. As a scientist, I was inexperienced at best; enthusiastic and curious, but very raw. Seeing as how I continue to be the youngest in the Miller Lab, and many times the only participating undergraduate, this feeling of unpreparedness was only magnified as all my peers seemed to have everything figured out.
For the first few weeks of the summer, I constantly felt behind and was more of a responsibility for my PI than a boon. I cannot overstate, however, how transformative the last few weeks have been for me.
After spending time with the PhD students in the lab, I began to stop viewing my situation as “the least experienced in the lab” and started to view it as "surrounded by intelligent scientists in my field." This distinction was a huge unconscious barrier to get past. It would not be an overstatement to say that this was the most important and humbling experience in my scientific life. Since overcoming my initial mindset (which was only possible thanks to the generous funding allowing me to stay on campus this summer), I have made leaps and bounds in both technical knowledge about my field and general know-how about being a scientist. I cannot thank the generous donors enough for this wonderful opportunity, as without it I would be half the scientist I am today.
Giving Back Reflection
As a bit of background, I was consistently the youngest and least experienced student in the Miller Lab. That is, until recently, when an undergraduate the same year as me expressed interest in the lab and started to attend lab meetings with the goal of figuring out whether computational neuroscience was right for him. Unfortunately, lab meetings end up being very jargony and complex, especially for an outsider.
Fortunately, I knew very well what it felt like to be the new undergraduate in a graduate lab. I take pride in the fact that I have been able to serve as a relatable mentor/peer figure. I will continue to encourage him to pursue computational neuroscience, despite its complex nature and confusing terminology that is foreign to newcomers.
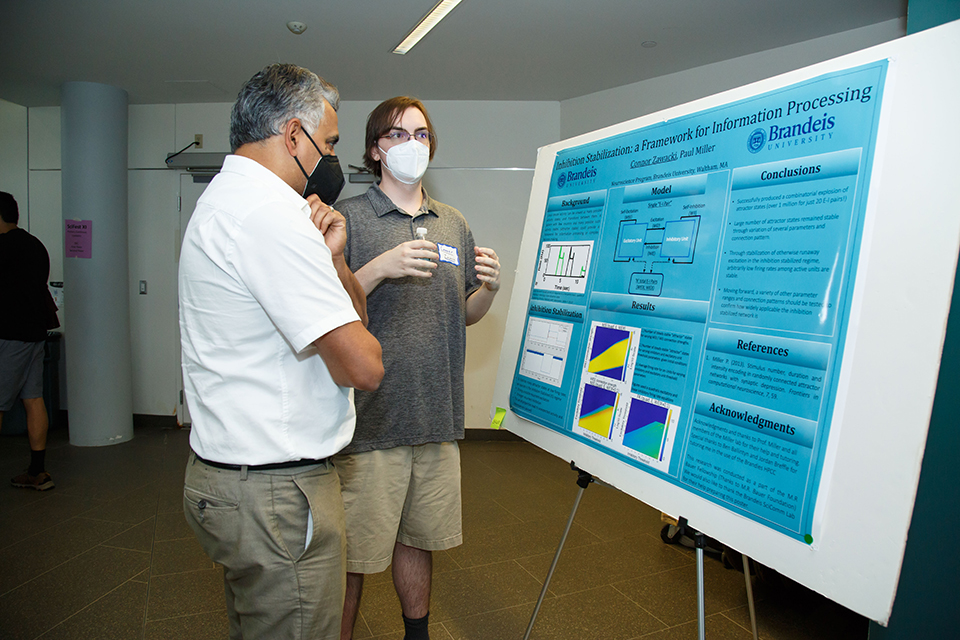